Sahar’s Coding with AI guide
How to treat your AI coding agent (Cursor/Windsurf/Cline) as a human pair programmer and yield the best results
Welcome to the first post in the AI Coding Series, where I'll share the strategies and insights I've developed for effective AI-assisted coding. In upcoming posts, I'll delve deeper into leveraging tools like Cursor and Windsurf, share best practices for developing secure AI applications, and more.
In this post, I’ll share the principles and tactics that helped me go from chaotic vibe coding sessions to consistently building better and faster with AI. Subscribe to get notified when the next post is published.
A NotebookLM-powered podcast episode discussing this post:
In today’s “vibe-coding” world, where tools like Cursor, Windsurf, Cline, and Claude Code can autocomplete an entire feature before your coffee cools, speed is no longer the bottleneck. Clarity is. Early on, I mistook these agents for magical refactor engines: give them a prompt, lean back, wait for perfect code. Instead, I got files rearranged beyond recognition, subtle bugs, and a creeping sense that I was pair-programming with an eager intern running on double espresso shots. Not great.
Through experimentation, failures, and continuous learning, I discovered a critical insight: treating your AI coding agent like a highly capable human pair programmer dramatically improves performance. Just as clarity and thoroughness are crucial when collaborating with human teammates, providing explicit context and structured guidance to AI coding agents is equally essential.
In this post, I’ll walk you through the principles and tactics that transformed my chaotic vibe coding sessions into a consistent, faster, and higher-quality AI-assisted workflow.
The first three tips are foundational. They’ll show you why a successful vibe coding session is roughly 80% planning, 20% execution.
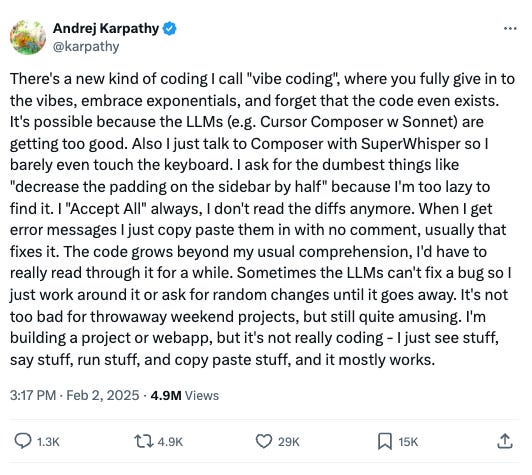
#1 Wear the Product Manager hat
The single biggest unlock I’ve found is to treat the project the way a seasoned PM would—before the first line of code is generated. A couple of hours of purposeful “spec-ing” gives the AI (and yourself!) all the needed context and saves you days of refactors later.
Create a Product Requirements Document (PRD)
Start every project with a prd.md file in the root directory explaining what you’re building, why, the user flows, in-scope / out-of-scope items, and a short tech-stack overview.
I have a PRD template I follow for my projects and you should have one, too. To save time generating it, I often use ChatGPT’s built-in Whisper to dictate my raw notes and then ask it to transform those notes into a PRD using my template. I also ask ChatGPT to surface any missing parts or context in my PRD before copy-pasting the output into the project’s prd.md file.
Then, add a rule to .cursor/rules or .windsurfrules (more on this below), encouraging the AI agent to “always read prd.md before writing any code”.
Break the knowledge base into bite-sized docs
For most side projects, one prd.md file is enough, but once it grows, split it:
app_flow.md - wire-frame-level tour of every page/endpoint and transitions from one page to another
db_schema.md - canonical source of truth for tables, relations, enums
tech_stack.md - chosen libs, versions, style guides, links to API docs
implementation_plan.md -step-by-step build checklist
Organize these files within a project-docs directory. Update your AI agent's rules to include:
Refer to all documents in the /project-docs directory for context before proceeding with code generation.
This modular approach provides your coding agent with the much-needed context to build your project correctly. I’ve consistently seen how a well-structured and documented project-docs directory leads to faster and better coding.
Feed the agent real artifacts
Drag-and-drop your Figma export, Swagger spec, or PDF PRD right into the chat (Cursor, Windsurf, etc. can ingest files). The richer the context graph, the fewer guesses the model makes.
Remember: the AI is a bright but literal teammate
I spend a whole day up front writing the product doc, test flows, and API contracts.
By adopting a product manager's mindset and providing comprehensive, organized documentation, you set your AI coding agent up for success. This structured approach minimizes misunderstandings and streamlines the development process, allowing you to build more effectively and efficiently.
Become a premium member to access the full LLM Builders series, $1k in free credits for leading AI tools and APIs (Claude, Hugging Face, Deepgram), and editorial deep dives into key topics like AI Voice Agents. It's also a great way to show your support :)
Many readers expense the paid membership from their learning and development education stipend.
#2 Planning with frontier models
As models proliferate (Gemini 2.5 Pro, Claude 3.5, o3, o4, GPT-4.1), developing intuition for which model to employ when becomes crucial for efficiency. Think of your AI stack like a toolbox. Different tools for different jobs.
Most developers, including myself, use different models for various phases of the development cycle. For the planning phase, leverage advanced reasoning models like Claude 3.7 Sonnet or o3 to write the PRD and generate implementation plans. These models excel at complex reasoning and can produce comprehensive, well-structured documentation that serves as the foundation for your project.
Recently, I discovered Anthropic's "ultrathink" technique: appending this keyword to your prompt signals Claude to allocate more thinking budget to your request. This results in more thorough plans at the expense of higher latency, which is a worthwhile tradeoff for critical planning sessions.
For instance, when recently developing an evaluation framework for voice agents, I used Claude 3.7 to architect the entire system. I prompted:
Based on these project requirements and the existing evaluation metrics in my /project-docs folder, generate a comprehensive implementation plan for building a voice agent evaluation framework that simulates conversations and scores performance across multiple dimensions. Include conversation flow design, metrics calculation logic, and reporting structure. ultrathink
The resulting implementation plan was exceptionally thorough. It proposed a conversation simulator with configurable user personas, identified seventeen distinct evaluation metrics (including non-obvious ones like 'recovery from misunderstanding'), suggested a weighted scoring system that accounted for business priorities, and outlined a modular architecture allowing for easy addition of new evaluation criteria as voice agents evolve.
After reviewing and refining the initial plan, addressing any missing pieces or gaps the LLM overlooked, I transition to different models for implementation: Gemini Pro 2.5 for most code generation, with Claude 3.5 and GPT-4.1 reserved for coding tasks that are local, e.g. generating/fixing a small function. Claude 3.7 and similar advanced models tend to overextend, introducing extra suggestions that require additional cleanup, so I avoid them for scoped changes.
#3 The building block approach - break tasks into atomic components
Rather than overwhelming yourself and your AI pair programmer with an entire project at once, think of your development process as assembling LEGO blocks. Each component should be well-defined, independently testable, and have clear inputs and outputs.
Start a fresh chat with your AI agent (⌘ + I) for each component to maintain focus and prevent context contamination. This approach not only helps the AI generate more precise code but also makes debugging and integration significantly easier.
For relatively self-contained components, I've found tremendous success using a separate development environment:
Open a new Cursor instance in an empty project directory
Copy only my project-docs folder into this environment
Ask the AI to build just that new component I need
Test the component thoroughly in isolation
Integrate the polished component back into the main project
A recent example from my voice agent evaluation platform mentioned earlier: I needed a component to transform raw JSON evaluation results into an interactive HTML dashboard displaying performance metrics. Rather than complicating matters by building within the already complex codebase, I isolated this visualization task entirely by generating the code in a new isolated environment and copying it back once done.
This isolation technique works especially well for visualization components, data transformation utilities, API clients, custom algorithms, and reusable UI elements.
Remember: the smaller and more focused the task, the higher the quality of the AI-generated solution.
The Open-Source Toolkit for Building AI Agents
Welcome to a new post in the AI Agents Series - helping AI developers and researchers deploy and make sense of the next step in AI.
#4 Use git for version control as a safety net
Git serves as an indispensable safety net, allowing you to track changes, revert to previous states, and understand the evolution of your project.
Tools like Cursor and Windsurf are powerful, but their change tracking interfaces are somewhat clunky, and as models get more aggressive about editing larger parts of your codebase, the more overwhelming such comparison interfaces will become.
Frequent commits act as checkpoints in your development journey. By committing often, you create clean, incremental snapshots of your project, making it far easier to review changes, spot unintended edits, and quickly revert mistakes.
What makes committing easier is Cursor’s and Windsurf’s built-in “Generate Commit Message” button. This feature analyzes your changes and automatically drafts a descriptive commit message, transforming what used to be a chore into a one-click process.
#5 AI IDE rules (.cursor/rules or .windsurfrules)
One of the highest-leverage moves you can make when working with AI coding agents like Cursor or Windsurf is to define clear, project-specific rules.
Think of rules as your agent’s operating manual: the clearer it is, the better your results.
At the start of every project, create a concise rules file, .cursor/rules or .windsurfrules, that guides how your AI pair programmer should behave. These rules act as a standing context layer that supplements every prompt, saving you from endless re-explaining and firefighting.
Here’s what great rules include:
Your project-specific guidelines such as “Always prefer strict types over 'any' in TypeScript”
Tech stack details - tell your agent what you're using: Flask, FastAPI, Supabase, SQLite, etc. so it stops making incorrect assumptions
Known pitfalls and fixes - add proactive corrections here if you notice recurring errors (e.g., Cursor defaulting to the wrong Python version)
High-level project overview - summarize the purpose, major functionalities, and key files (you can link to your prd.md here, see previous section).
Use .md reference files alongside rules for better modularity. For instance, we once spent a full day as a team designing our testing philosophy, captured it in test-guidelines.md, and added a simple rule: “Refer to test-guidelines.md when writing tests.”
Another rule that dramatically boosted my high-stakes coding sessions:
For any complex or critical task, ask any and all clarification questions needed before proceeding.
Cursor recently introduced a /Generate Cursor Rules command, allowing you to instantly create new rules based on the current chat context, which is particularly useful after a significant architecture change or a project refactor.
Extra resources:
Browse and reuse existing community rules: Cursor Directory and Playbooks Rules
Build your own rules easily: Playbooks Rule Builder
#6 Generate an MCP server for any API in two commands
Keep reading with a 7-day free trial
Subscribe to AI Tidbits to keep reading this post and get 7 days of free access to the full post archives.